The AI in Education Summit was an all-day, in-person, invitation-only gathering of around 80 participants from across education, technology, the charitable sector and government. It was part of the AI Fringe inspired by the UK government's global AI Safety Summit.
Prior conversations with dozens of people revealed huge enthusiasm for a meeting that would:
- Foster communication across the often separate silos of education, technology and government in order to share diverse experiences and viewpoints
- Provide a low-risk environment in which to express emerging ideas, nagging concerns and contentious speculations
- Adopt an expansive definition of AI that goes beyond chatbot tutors into areas such as adaptive assessment, predictive analytics and curriculum design
- Adopt a similarly broad view of education that encompasses not only compulsory schooling and higher education, but also self-study, professional development and lifelong learning
The discussion was lightly structured and curated, with loosely themed sessions and brief thought-provokers to kick things off, but the main emphasis was on freeform debate in order that everyone could benefit from each other's perspectives.
The event was conducted under the Chatham House Rule, but we are pleased to share anonymised summaries of the conversations below.
A message to participants π§
Thank you to everyone for making the Summit so informative, stimulating, challenging and fun. Many of you have expressed your appreciation to the organisers, but you should really be thanking each other: you're a remarkable bunch of people. We hope you find the summaries below useful. You can continue the conversation on the Summit Discord server, which you can also use to send us feedback via the #post-summit-feedback channel. If you have further questions or requests then please send us an email: hello@edsummit.ai. π
Plenary sessions π¬
These used the following framework, with three broad areas of discussion: How We Learn, What We Learn and How We Work:
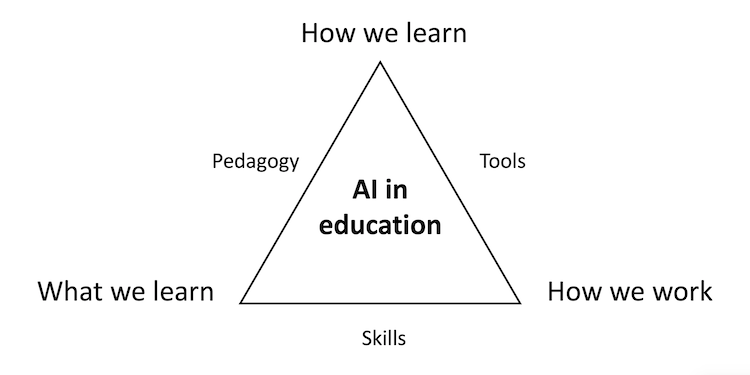
The wide-ranging discussions were captured in visual form by our scribe, James Baylay, in the image work of art below. You can use the circular 'magnifying glass' to zoom in to particular parts of the picture, but much better to open it in a separate tab or window – preferably on a big screen. π
(Click here to view the image above in a separate tab or window. Even higher-resolution versions are available on request – write to: hello@edsummit.ai.)
We also used Padlet to collect notes and comments from participants while the conversations were taking place and then fed these into (what else?) ChatGPT to extract the major themes. Inevitably, given the breadth of the topic and wide range of views, both graphical and text summaries are rather impressionistic and subjective. But as befits the subject, these crowd-sourced, AI-enabled summaries seem to complement the human, hand-crafted overview above. Here are the results:
How We Learn π§βπ
- Human Involvement: Emphasizing the need to keep humans in the loop in AI applications, questioning what form this involvement should take, especially in monitoring student progress.
- Balance and Evidence: Concerns about premature claims of AI's benefits in education, akin to the wellness industry, with a call for more oversight and evidence-based claims.
- Educational Consumers of AI: Highlighting the need for educators, institutions, and governments to become more sophisticated consumers of AI, possibly through trusted frameworks or certification to help make informed decisions.
- Innovation vs. Government Funding: Debating whether government-funded AI stifles market innovation, and the challenges of AI investment in education due to high costs and lack of a clear business case.
- AI and the Curriculum: Questions about the reliability, pedagogical soundness, and ethical considerations of AI in curriculum alignment and teaching.
- Regulation and Risks: Discussing the necessity and extent of regulation in AI for education, considering the high-risk classification of AI applications in education by the EU.
- Evidence and Innovation in AI: The idea of innovation-led evidence versus evidence-based innovation, emphasizing the need for satisfactory evidence at the start of AI applications in education.
- Diversity in AI Approaches: Recognizing that different AI systems have varied strengths and weaknesses, and not all AI solutions are the same.
- AI Adoption by Young People: Noting that young people are already engaging with AI in informal settings, raising concerns about safety and the need to align formal education with these experiences.
- Standards and Safety in AI: The potential need for technical standards like ISO for AI in education, to ensure safe and effective deployment.
- Social Nature of Learning and AI: The challenge of AI supporting collaborative and collective learning activities, and the roles involved in social learning.
- AI's Role in Addressing Disadvantage: The importance of considering access to AI among disadvantaged groups, and the ethical considerations in the use of data and technology in education.
What We Learn π
- Importance of Traditional Knowledge: Emphasizing the value of traditional subject areas as a medium for developing advanced human skills, with AI augmenting but not replacing this knowledge.
- Societal Change and Education: Stressing the need for educational thinkers to consider the broader societal changes and how AI can catalyse existing trends like micro-credentials and lifelong learning.
- Equity and Access in AI Education: Highlighting the risk of exacerbating the digital divide and the need to ensure that AI in education doesn't worsen existing inequalities.
- Curriculum Reform with AI: The necessity of redesigning the curriculum to emphasize human skills, as AI takes over more computational and data-driven tasks.
- Teaching Knowledge and Skills: Balancing the teaching of traditional knowledge with the development of skills like critical thinking, creativity, and problem-solving in an AI-integrated curriculum.
- Data Literacy: The importance of teaching students not just how to find data but also how to understand its creation and context, fostering a healthy scepticism.
- Qualifications and Employment Relevance: Ensuring that qualifications remain meaningful and relevant to employers in an AI-influenced job market.
- Rethinking National Curriculum: Questioning the purpose of the national curriculum in the current educational landscape and considering the need for more functional skills qualifications.
- Future Skills and Technology: The challenge for governments and educational institutions to anticipate future skills needs and integrate technology effectively.
- Critical Thinking and Information Literacy: The imperative of teaching critical thinking and information literacy from an early age to cope with the influx of AI-generated information.
- Trust and Knowledge Evaluation: Cultivating students' ability to critically evaluate sources and trust the information, separating practical application from technical understanding.
- Role of Humans in AI Era: Defining and understanding the evolving role of human teachers and learners in the age of AI, particularly in teaching complex skills like creativity and critical reasoning.
How We Work π©π»βπ»
- AI in Marking Systems: AI can detect and delete blank sheets, aiding marking systems, but struggles with actual grading.
- Problems with Generative AI: Challenges include "hallucinations" (making things up) and stability (inconsistent answers). Context matters in assessing the impact of these issues.
- Use of AI in Teacher Training: AI can simulate classroom situations for role-playing in teacher training, focusing on improving pain points rather than replacing activities.
- Impact of AI on Work and Learning: AI is revolutionizing learning material creation, skill assessment, career trajectory suggestions, and compliance management, raising questions about lifelong learning and reskilling.
- Challenges of Implementing Change: Difficulty in creating a culture of change in educational and commercial organizations.
- Collaborative Work in Education: Need for rapid scaling of successful collaborative experiments to avoid negative impacts on education and the economy.
- Evaluating EdTech Quickly and Robustly: Balancing the need for robust evaluation with the speed of technology development.
- Safeguarding and Framework Consistency: Establishing consistent frameworks for human interaction with AI systems, focusing on safe and human-centred approaches.
- Commercial Models and Development Pace: Balancing the needs of developers and end-users, with examples like Khanmigo showing potential but lacking independent assessment.
- Shared Evidence Frameworks: Creating incentives and frameworks for excellence in educational technology, visible and comparable across institutions and countries.
- Focus on Harm Prevention: Emphasizing not only what works in education technology but also what doesn't cause harm.
- Centralised Signposting and Research Accessibility: The need for centralized mechanisms to connect startups with problem owners, and for open data and broader data sharing to enable further research.
Unconference sessions π‘
During the afternoon, there were a number of 'unconference' breakout sessions on subjects suggested by participants and decided on the day. Here are brief ChatGPT-generated summaries:
How will we know if AI is safe and beneficial? β’οΈ
- Framework for Data Use: Need for a framework that respects the rights and agency of children while making data available for the public good. This includes considerations of privacy, education, agency, and wider societal impacts.
- Lack of Vision in EdTech: Many educational technology (EdTech) solutions are developed without a clear educational vision, focusing more on technological capabilities than educational outcomes.
- Macro Risks and Heterogeneity in Education: Importance of considering the broader risks of AI in education, not just its immediate effects in a lab or classroom. Recognizes the complexity and diversity of education systems and the challenge in measuring improvement.
- Data Ownership and Use: Data should not be owned by the providers of EdTech systems. Need for careful consideration on how to use it in the best interests of the child, which is beyond the capacity of individual schools.
- Comparative Insights and Data Access Dilemmas: Lessons from other sectors, like health, and different countries. The challenge of balancing data access for analysis with privacy and efficacy, citing examples from the Netherlands and Denmark.
- Assuring Appropriate Use of Technology and Data: Concerns about how schools and users can ensure that technology and underlying data are not misused. It points out the challenges faced by companies in implementing best practices and the necessity for clear terms of use, especially in differentiating consumer versus enterprise services. The role of digital watermarks in verifying the authenticity of content and data.
What do we want our relationship with AI to be? β€οΈ
- Clarity and Regulation: There's a concern about the lack of clarity on what we want from AI, making it challenging to establish effective regulations. This is particularly significant in the context of education, where the potential for both utopian and dystopian outcomes exists.
- Cognition and Dependency: There's a worry about offloading our cognitive abilities to AI, as seen in examples like using satellite navigation systems instead of maps. This could lead to a decline in human cognitive skills.
- Humanity's Future vs AI's Future: The discussion shifts focus from the future of AI to the future of humanity itself, emphasizing that the use of AI in education should be more about enhancing human capabilities and wellbeing rather than just technological advancement.
- Human Interaction and Dehumanization: There's a concern about AI potentially dehumanizing us, especially in education where students might prefer interaction with AI like chatbots due to their friendly and non-judgmental nature.
- AI's Role in Education and Society: AI is seen as a tool to prepare students for the future workforce, especially in jobs with a social purpose. This includes a debate on whether AI should primarily aim to increase human intelligence or wellbeing.
- Engagement and Decision-Making: The need for broader engagement in discussions about AI's role in society is emphasized, suggesting methods like Citizens Assemblies. Also, there's an exploration of how AI can be used to assist in learning and decision-making without overtaking human control.
What works and what doesn't (yet)? π§
- Assessment Challenges: AI has been used to identify blank pages and recognize ticks and crosses in scripts, but struggles with handwritten assessments, showing only a 78% reliability rate. This issue isn't unique to this AI but is common across the industry.
- AI in Grading: Attempts to use AI for grading, including with GPT-3 and GPT-4, have been underwhelming. Issues include unreliable result clustering and poor consistency when re-running the marking on the same pieces of work.
- Inconsistencies and Hallucinations: AI models, being probabilistic, tend to "hallucinate" and produce varied results for the same prompts. This leads to inconsistencies in marking compared to human assessments.
- Resource Generation and Helpdesk Support: AI has not been successful in generating personalized educational resources or functioning effectively in helpdesk roles, often producing erroneous responses.
- Human Oversight is Crucial: Despite technological advancements, human intervention remains necessary in all aspects, from marking to resource creation. The effectiveness of AI needs to be weighed against the time savings it offers.
- Limitations in Handwriting Recognition and AI-generated Essays: Handwriting recognition by AI is not yet reliable for diverse styles. Furthermore, while AI can sometimes produce essays that pass moderation, it's not reliable enough for marking or identifying AI-generated "cheat" essays.
How do we get subject change? ποΈ
- Integration of Computational Thinking: There was a focus on incorporating computational thinking into the curriculum, with suggestions to revise the current maths curriculum significantly.
- Realistic Prospect and Curriculum Placement: Debates centred around how realistic this integration is and where computational thinking would best fit within the current curriculum, both in terms of student age and relevant subjects. Targeting the new 16-18 βappliedβ or βpracticalβ maths curriculum was discussed.
- Role of Universities and Employers: The influence of universities and employers in shaping this educational change was considered. However, it was concluded that they might not clearly understand their needs or be the best initiators for such changes.
- Political and Communication Strategies: The importance of influencing key policymakers and government officials was noted, especially in the context of changes in government and education influencers.
- Pilot Programs in Schools: The suggestion of using pilot programs in independent schools, which are reducing GCSEs to free up curriculum time, was mentioned. Additionally, KS3 in state schools was identified as a flexible period for testing aspects of the new curriculum, as there are no public exams at this stage.
- Recruitment of Enthusiastic Maths Teachers: The strategy includes recruiting enthusiastic maths teachers, particularly at the KS3 level, to trial elements of the new computational thinking curriculum.
What would it look like to use AI to teach critical thinking? π€
- Rise of Critical Thinking in Education: The importance of critical thinking in public education, focusing on sceptical analysis, reasoning, and understanding scientific methods. The need to recognize and overcome intrinsic biases like confirmation bias.
- AI's Role Amidst Information Overload: The growing importance of critical thinking in an era of information overload and misinformation, questioning how AI, often unreliable, can contribute to this.
- Chatbots and Socratic Dialogues: The use of chatbots in creating psychological illusions, like the 'Eliza Effect', and their potential in engaging in Socratic dialogues, taking a 'devil's advocate' stance to stimulate critical thinking.
- AI and Educational Assessment: Assessing critical thinking, which is traditionally reduced to essay writing, and the role of generative AI to provide answers while potentially bypassing the critical thinking process.
- AI as a Teaching Aid vs. Teacher-Led Exercises: Could AI supplement areas where schools lack skills and capacity, particularly in comparison to teacher-led exercises, and might reliance on AI reduce personal capabilities, akin to the effect of using GPS.
- Broader Approaches and Involvement in Critical Thinking: Using AI to assist students in expanding their own thinking and research, promoting holistic thinking, and integrating approaches like video games for experiential learning on topics like confirmation bias. Involving children and young people in these discussions.
How is the balance of power in education shifting? πͺ
- System Change and Disruption: AI technologies are significantly altering the balance of power in education, calling for a system-wide change. This involves managing shifts in power dynamics due to the disruptive nature of these technologies.
- Acceleration of Instructional Material Creation: There's a rapid increase in the creation of personalized instructional materials using AI, especially in text and audio formats. These materials are quicker to produce and can be highly specialized, though they might still require human oversight.
- Shift in Content Production: Schools, with their rich student data, might become better at producing and distributing instructional materials than traditional publishers. This shift raises questions about the roles of different entities in content creation and distribution.
- Market Dynamics and Production Costs: Lower production costs are leading to new market entrants, altering the traditional landscape. The discussion explores whether schools and other entities will choose to produce their own materials based on factors like quality, cost, and time.
- Discipline-Specific Variations and Comprehensive Resources: There are notable differences in the availability and nature of AI-generated instructional materials across various disciplines. Additionally, the integration of these materials with data about learning progress is still an evolving area.
- Data Control and Power Dynamics: Concerns about a few large, non-transparent entities gaining control over educational data and resources, similar to the situation with learning management systems provided by major corporations. This control could impact the access to and use of educational data.
Human-generated text by Timo © 2023